LOD Link Discovery
A commonly used representation of semantic enriched data is Linked Open Data (LOD) - data should be structured and made available in an open and non-proprietary format. Furthermore, resources are described by dereferencable HTTP URI’s and interlinked with other URI’s. Within the LOD Link Discovery project, we investigate techniques to improve the quality of the LOD data sets - either by creating new links or by improving the quality of already existing links. Context and relations between entities are exploited as well as holistic approaches for Link Discovery, e.g., employing multiple data sources. These techniques are complemented by methods improving the scalability of Link Discovery - parallelization via Hadoop cluster enables the use of MapReduce, In-Memory computation and graph processing systems.
Holistic Clustering on Linked Data
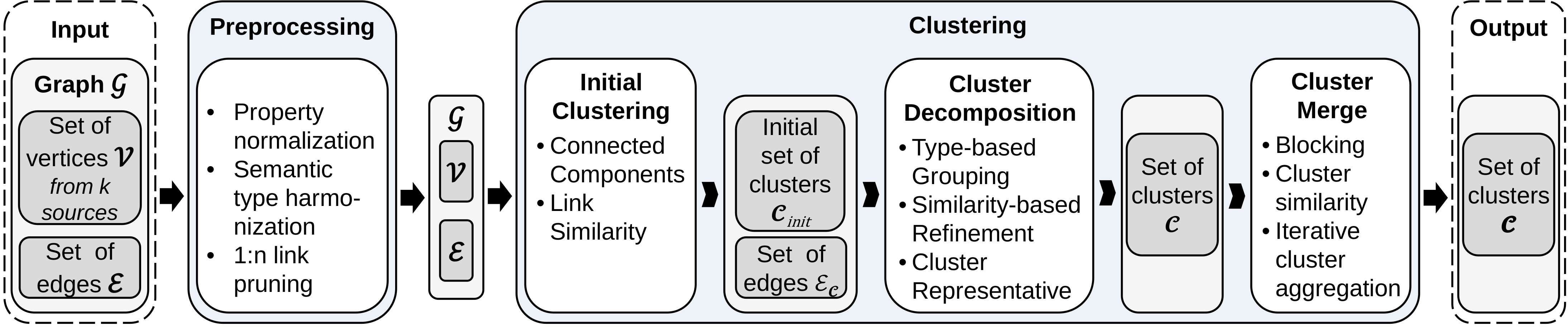
Reference Dataset for multi-source clustering in the geography domain
We provide a manually curated reference dataset for multi-source clustering to
support the evaluation of holistic clustering approaches w.r.t. the quality of generated
clusters. The dataset as well as the curated clusters can be downloaded here.
People
Partners
Funding / Cooperation

Publications
|